Sex and Gender Bias Considerations for Data Science
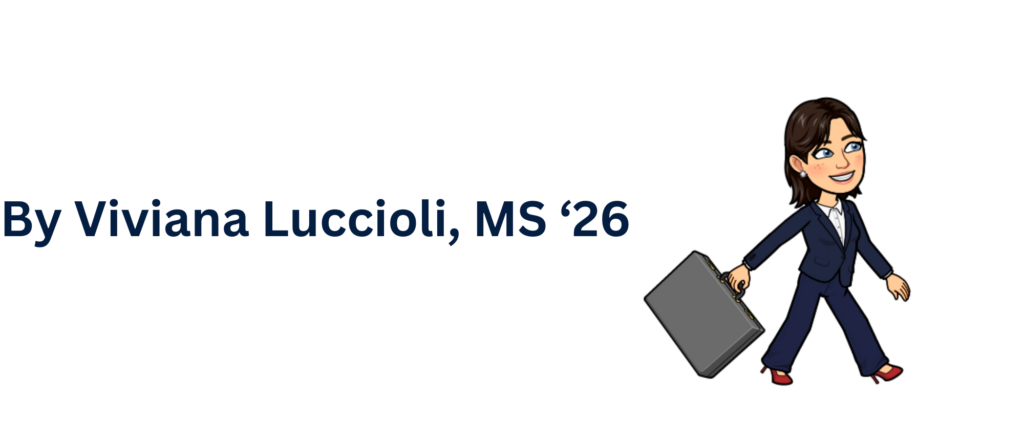
When I was young, I thought it was so unfair that my left-handed friends struggled when using scissors or had to go out of their way to sit at one of the few left-handed desks in the classroom– and when they weren’t available, they had to deal with being uncomfortable. I couldn’t believe it! The world wasn’t designed for them, and in attempts to adapt, they faced discomfort and disadvantages.
I later understood that from a logistical standpoint, systems had to have fewer amenities for left-handed people because they make up approximately 10% of the population. They are a minority.
As I grew up, I started to think about how women make up half of the population, so they aren’t a minority (quantitatively.). So then why do they live in a world that isn’t designed for them, facing discomfort and disadvantages when trying to adapt?
I recently read Invisible Women: Data Bias in a World Designed for Men by Caroline Criado-Perez because I wanted to enter my data science education with an informed foundation of the sex and gender data gaps. Data scientists are the “authors” of artificial intelligence. As a whole, we possess the power to control what the rest of the world knows and is able to accomplish from AI– and with great power comes great responsibility. In a world where AI is increasingly becoming a tool that people rely on for help, explanation, and even the substitute for peoples’ jobs, it is imperative that the information AI produces reflects truth. The most basic data consideration is that data should be representative of the population. Not only by the design of those who have historically and systematically been more advantaged to land careers in data science.
As a quick refresher, “Bias in data is an error that occurs when certain elements of a dataset are overweighted or overrepresented. Biased datasets don’t accurately represent ML model’s use cases, which leads to skewed outcomes, systematic prejudice, and low accuracy. Often, the erroneous result discriminates against a specific group or groups of people.” 1 Machine learning (ML) and AI have the potential to amplify existing biases.
The main problem here is not that systems are purposefully designed specifically out of spite against women, but that women are simply not included enough in data that is used to inform important things, or in enough of the choices and design processes of our current public AI models. Estimates claim that women only make up 22% of AI and data science professionals2, and this is a big problem because it means that the majority of AI and data science models are created with hardly any female perspective or input. And although the number of women in the data science field is increasing, it doesn’t inherently reverse the accumulation of hidden biases so ingrained in our daily lives that we don’t even recognize them. It’s important to learn about them so that we can understand how to narrow the various channels of the sex and gender gaps moving forwards.
Invisible Women walks readers through how the male default bias, which refers to how typical masculine characteristics, ideologies, physiologies and perspectives are treated as the “standard” while the same premises for non-males are considered the “other,” both contributes to and perpetuates the gender data gap. The gender data gap describes the lack of non-typical-male representation in data that informs systems, models, and future research. Since the male default follows that what is male is normal and what is not is a deviation, it assumes that basing data off of mainly typical men is sufficient for the needs of everyone. But it’s not.
Female bodies, experiences, and needs are not the same, and this leaves many systems and models tuned for men and uncomfortable and disadvantageous for women.
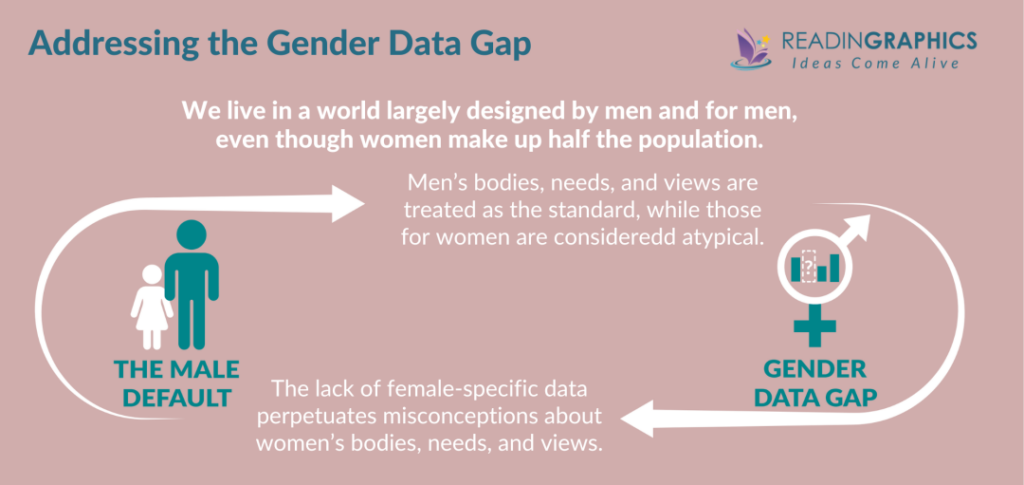
There were four themes discussed in Invisible Women that stood out to me the most as considerations for data science: data bias ingrained in 1.) large language models and translation AI, 2.) clinical trials and public health, 3.) safety, and 4.) urban planning.
- Large Language Models and Translation AI
Research has shown and practice has suggested that LLMs are trained on imbalanced datasets, and they tend to reflect those imbalances back, even with recent advancements in reinforcement learning with human feedback. A famous study found that LLMs “followed gender stereotypes in picking the likely referent of a pronoun: stereotypically male occupations were chosen for the pronoun he and stereotypically female occupations were chosen for the pronoun she”, and that “LLMs amplified the stereotypes associated with female individuals more than those associated with male individuals.”3 A classic example is that in Turkish, a gender-neutrally structured language, “o bir doktor” translates to “[any pronoun] is a doctor.” However, Google translate used to output “he is a doctor” when prompted for that translation.4
In 2018, Google released a statement announcing, “we’re taking the first step at reducing gender bias in our translations. We now provide both feminine and masculine translations when translating single-word queries from English to four different languages (French, Italian, Portuguese, and Spanish), and when translating phrases and sentences from Turkish to English.”5 The first step came a bit late, and the work being done is still not sufficient, for she and he are not the only gender pronouns.
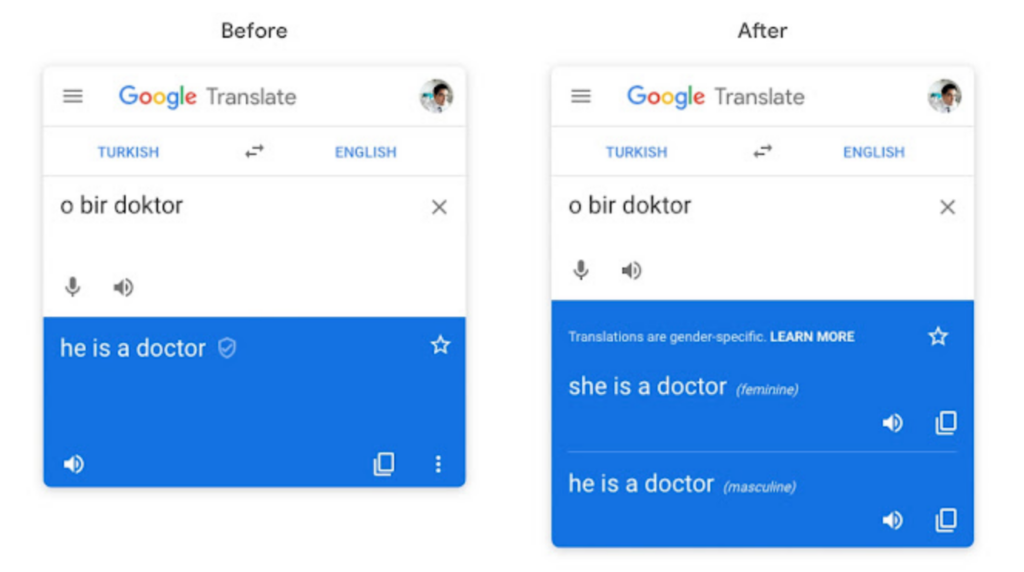
The world is changing and growing. Though there still exists a significant disparity, women and nonmales are increasingly taking up space in professional fields that were historically predominantly male. The LLMs we develop should reflect the future, not be stuck in the sexist past.
- Health & clinical trials
Women are disproportionately underrepresented in clinical trials.6 Therefore, claims like “this drug is 95% effective” are not always true– they’re only really true for men.
Gender data bias explains why Apple’s health tracker app did not include a menstrual cycle tracker when it launched, a feature that can be easily estimated and immensely helpful for a large proportion of users. This is a failure on the part of the data scientists and app developers. It also explains why in the U.S. there are five approved oral medications for erectile dysfunction, but none specialized for menstrual pain (we are just told to apply hot water and take an Ibuprofen). So apparently in clinical research, sexual pleasure for men appears to be more important than debilitating, excruciating pain experienced by women. It explains why Teva Pharmaceuticals faced lawsuits for marketing and manufacturing ParaGard IUDs that they knew could cause serious harm to people who use them.7 Do you think this product ever would have been released had the patients been men? There needed to be more honest data science and analytics before the release of this device, and this stresses the importance of data scientists having ethical backbones.
But beyond the gender data gap, there is an even deeper problem– the racial gender data gap. Though the breast cancer mortality rate among Black women is 41% higher than that of white women8, Black women only make up 5% of participants in clinical trials9. Black women are also three times more likely than white women to experience a pregnancy related death.10 These two examples make up a tiny fraction of the health disparities experienced by Black women, which are perpetuated by their lack of inclusion in data and subsequent lack of information and prioritization in healthcare. It is important to note that increasing data inclusion of only certain subgroups of women, presumably white women, could actually do the opposite of leveraging equity– it could drive further disparities and systematic disadvantages among minorities.
- Vehicle safety
When car companies say that their products are safe, can we be sure they are truly safe for all? Women have historically been disproportionately injured and killed in car crashes, and that is because the safety of original car models were designed on male dummies only. A study that after 2003, when the vehicle safety testing standard had been changed to mandate the use of a female dummy in an occupant protection for front-impact testing, women were 19.2% more likely than men to be severely injured from crashes, compared to being 41% more likely before 200311. Including women in the data that we use to inform important features proves to save many lives and hospital bills.
- Urban planning: can snow clearing be sexist?
“Can snow clearing be sexist?” was one of my favorite sentences in Invisible Women. When I read it, I almost laughed and thought, “no way!” Snow clearing procedures tend to first clear major roads, and then work their way down to smaller ones. The book notes that, typically, women spend more time on the smaller roads– pushing strollers, taking children to local activities, and running errands for other forms of unpaid labor.12 A study in Sweden investigated if changing the snow clearance procedure would have any impact on road injuries in the winter, and it found that “79% of pedestrian injuries occurred in winter, of which 69% were women. The estimated cost of these falls was 36 million Swedish Krona per winter, about USD $3.7 million USD. By clearing paths first, accidents decreased by half and saved the local government tons of money.” These findings validate that considering womens’ typical routes and daily routines when planning snow clearing priorities had significant public health and economic benefits.
I strongly encourage you to read this book because I believe it will expand your perspective. And I didn’t write this blog to only motivate you to consider gender bias in your future with data, because gender is only part of the systematic bias problem. Race, ethnicity, LGBTQ+ identity, age, poverty and more are all subject to being disproportionately represented in the technology that may soon have an even more powerful chokehold over our global society. There are so many different types of biases present, so many that we don’t see or realize being ingrained in the models from which we train and design powerful technology and intelligence.
We must always remember that data isn’t fact. Data is potential fact that goes through the hands of subjective, individual humans, who subconsciously and maybe even innocently may have their own biases. The output can sometimes be a slight deviation from fact. It’s a fact for some, but not all. As data scientists, we owe it to the world to provide honest, fair information and systems from the data we work with. As data scientists, we owe it to the world to represent all. To do this, we need representation from all in the data science field.
Aside: So women are like left-handed people, left to make do with tools that are not designed in consideration for them. Evidence has suggested, however, that left-handed people tend to be more creative and brilliant… 😳
To read next:
- Weapons of Math Destruction: How Big Data Increases Inequality and Threatens Democracy
- Algorithms of Oppression: How Search Engines Reinforce Racism
- Automating Inequality: How High-Tech Tools Profile, Police, and Punish the Poor
- 1 Kamińska, “8 Types of Data Bias That Can Wreck Your Machine Learning Models.” 2 Young, Wajcman, and Sprejer, “Where Are the Women?: Mapping the Gender Job Gap in AI. Policy Briefing: Full Report.” 3 Kotek, Dockum, and Sun, “Gender Bias and Stereotypes in Large Language Models.” 4,5 Johnson, “Providing Gender-Specific Translations in Google Translate.” 6 Bierer et al., “Advancing the Inclusion of Underrepresented Women in Clinical Research.” 7 Cochran, “Paraguard IUD Lawsuit Settlement Amounts (2024).” 8 Richardson et al., “Patterns and Trends in Age-Specific Black-White Differences in Breast Cancer Incidence and Mortality – United States, 1999–2014.” 9 Fernandes et al., “Enrollment of Racial/Ethnic Minority Patients in Ovarian and Breast Cancer Trials.” 10 Montalmant and Ettinger, “The Racial Disparities in Maternal Mortality and Impact of Structural Racism and Implicit Racial Bias on Pregnant Black Women.” 11 Fu, Lee, and Huang, “How Has the Injury Severity by Gender Changed after Using Female Dummy in Vehicle Testing?” 12 Criado Perez, Invisible Women: Data Bias in A World Designed for Men.